Food and beverage manufacturers can use predictive analytics to reduce product waste and downtime.
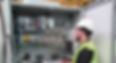
Unplanned downtime is one of the greatest barriers to cost and energy efficient food production. Failures or breakdowns of machinery can result in the need for unplanned repairs, unscheduled equipment maintenance and significant product waste. According to research by the Waste and Resources Action Programme (WRAP), up to 9% of waste produced in the processed food sector can be attributed to machinery problems like product blockages and mechanical mishandling.
Downtime should be avoided at all costs, particularly in a sector as energy- and cost-dependent as the food and beverage industry. That’s why manufacturers have, for a long time, implemented scheduled maintenance plans. Traditionally, these have entailed the implementation of annual or six-monthly schedules to replace and service machinery on the factory floor.
But these are not always the most cost-effective options. Furthermore, due to improper maintenance work, such plans have the potential to introduce faults where, previously, there were none. Instead, a better and more cost-effective solution can be found in software that is designed specifically for manufacturing processes.
Monitoring health
Manufacturers frequently overlook the importance of digital tools in favor of focusing on hardware – even though software has now evolved to a point where it can provide constant insights into the health of equipment.
Rather than inspecting machinery on a scheduled basis, simply because a timetable advises them to, food and beverage manufacturers can instead use this technology to repair items that actually need attention. Meanwhile, devices that are in good working order can remain in operation.
There are various forms of condition monitoring, many of which are enabled by sensors and these enable monitoring of vibration, pressure and temperature readings, which can help identify when a machine or part is beginning to show signs of wear. Advances in industrial automation software also enable better visualization of this information, so it is possible to react more quickly and with better insight — even across multiple sites.
Amalgamating operating information from various islands of data on the factory floor – be they from large machines or smaller components –provides a factory-wide view of performance. Using this information, it is possible to identify when a piece of equipment is showing signs of wear, and to act accordingly.
Such a solution can help reduce product waste. A recent study by Brunel University London and Ghent University which surveyed 47 Belgian food manufacturers found that, on average, they suffered a loss of one tonne of food for every 35 tons produced. The real economic cost of food loss within these food companies could be as high as 4% of turnover.
Elsewhere, WRAP’s report cites the example of Morrisons supermarket and Kerry Noon, the partner that supplies its ready meals. By improving working processes, the companies achieved a projected 7%, or 421 tonnes, reduction in ready meal waste. These savings were valued at £100,000 per annum.
Fortunately, in instances where such losses are caused by faulty machinery, software is available which can identify and address these problems. Machine learning technologies offers data models that inform when and why a machine might fail, based upon trends in machine data.
We can apply this example to the use of a food depositor. Depositors are often used in conjunction with belt conveying systems, to automatically fill product cases with an ingredient or mixture. Due to the combined effort of these processes, it is essential to ensure that the equipment maintains a speed that is synchronized with the conveyor.
Monitoring software can pinpoint the typical lifespan of the depositor’s motor by examining historical data from other equipment of this type. So, by combining this information with the typical operating hours of the factory and the production line speed, there is enough information to predict how, why and when the equipment is likely to fail or deteriorate.
Failure to identify a potential breakdown of the depositor could cause significant downtime. However, even the slightest reduction in efficiency might also skew the accuracy of depositing and cause faulty batches in production.
This method of condition monitoring is often referred to as predictive analytics. However, an influx of artificial intelligence (AI) developments is making this technique even more effective and advanced, which has led to the coining of the term “prescriptive maintenance”.
The right knowledge
The right software offers the ability to glean data from the Industrial Internet of Things (IIoT), like interpreting readings from sensors and actuators, and detect correlating patterns in equipment failure. By combining this data with historical and real-time data streams, manufacturers can gain enough knowledge to better tackle, or completely eliminate, unexpected breakdowns.
In this case, knowledge means gaining a basic understanding of why downtime and waste occur. Then, for the next step, analyzer software makes it possible to drill drown to the factors that are causing the losses, whether this is insufficient machinery performance or sub-optimal inventory management.
Traditional, planned maintenance will always have a place in food manufacturing. However, looking to the future, manufacturers have the opportunity to deploy technologies to inform, as well as act, when maintenance conditions arise.
This article originally appeared on Control Engineering Europe’s website.